「Zipf Distribution Model for Quantifying Risk of Re-identification from Trajectory Data」
2016年度論文賞受賞者の紹介
Zipf Distribution Model for Quantifying Risk of Re-identification from Trajectory Data
[Journal of Information Processing Vol.24 No.5, pp.816-823]
[論文概要]
In this paper, we propose a new mathematical model for evaluating a given anonymized dataset that risks being re-identified. Many anonymization algorithms have been proposed in the area called privacy-preserving data publishing (PPDP), but, no anonymization algorithms are suitable for all scenarios because many factors, e.g., a requirement of accuracy, a domain of attributes, a size of dataset, and sensitivities of attributes, are involved. In order to address the issues of anonymization, we propose a new mathematical model based on the Zipf distribution. Our model is simple, but it fits well with the real distribution of trajectory data. We demonstrate the primary property of our model and we extend it to a more complex environment. Using our model, we define the theoretical bound for reidentification, which yields the appropriate optimal level for anonymization.
[推薦理由]
匿名化されたデータの再識別リスク評価問題に対して、Zipf分布に基づく新しい数学的モデルを適合させて理論的な解析を可能にしており、データ活用とプライバシー保護を両立するために不可欠な匿名化技術に関する優れた論文である。
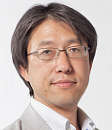
Hiroaki Kikuchi 君
He received his B. E., M. E. and Ph. D. degrees from Meiji University in 1988, 1990 and 1994.
After working in Fujitsu Laboratories Ltd. from 1990, in Tokai University from 1994, respectively, he joined Meiji University in 2013.
He is currently a Professor at the Department of Frontier Media Science, School of Interdisciplinary Mathematical Sciences, Meiji University.
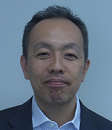
Katsumi Takahashi 君
He received a B.S. in mathematics from Tokyo Institute of Technology and a Ph.D. in information science and technology from the University of Tokyo in 1988 and 2006.
He joined NTT in 1988 and has studied information retrieval, data mining, security, and privacy.
He is an executive research scientist of NTT Secure Platform Laboratories.